Practical Applications of AI in Business: Beyond the Hype
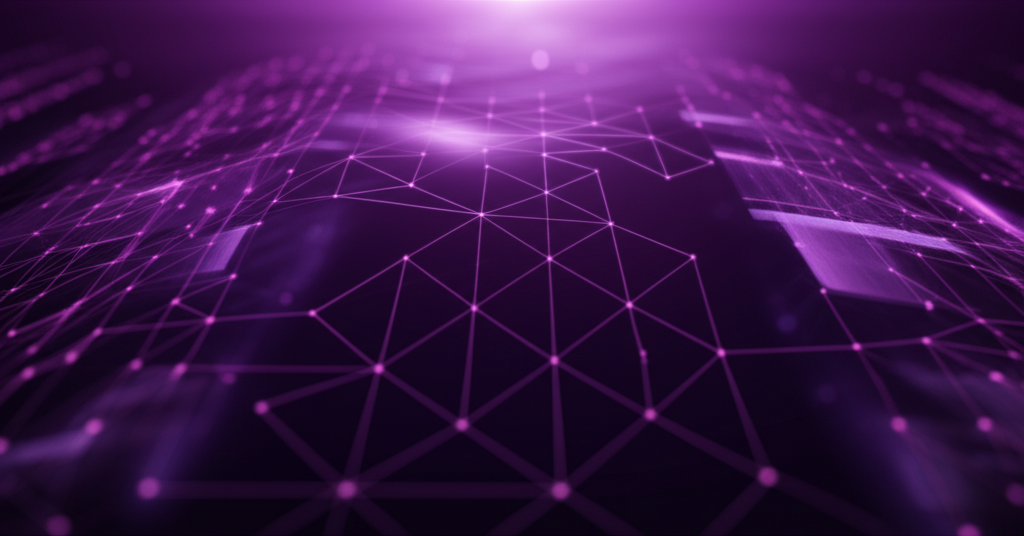
Artificial Intelligence (AI) has moved beyond buzzword status to become a transformative force in business operations. While there's still plenty of hype surrounding AI technologies, many organizations are now implementing practical AI solutions that deliver tangible benefits. This article explores real-world applications of AI in business, focusing on implementations that are creating value today.
Customer Experience Enhancement
One of the most mature areas of AI application is in customer experience:
Intelligent Chatbots and Virtual Assistants
Modern AI-powered chatbots have evolved far beyond the simple rule-based systems of the past. Today's conversational AI can:
- Understand natural language with high accuracy
- Maintain context throughout conversations
- Handle complex customer inquiries
- Seamlessly escalate to human agents when necessary
Case Study: A telecommunications company implemented an AI chatbot that now handles 70% of customer inquiries without human intervention, reducing wait times by 85% and increasing customer satisfaction scores by 23%.
Personalization at Scale
AI enables businesses to deliver personalized experiences to millions of customers simultaneously:
- Product recommendations based on individual preferences and behaviors
- Dynamic content customization
- Personalized email marketing
- Individualized pricing strategies
Case Study: An e-commerce retailer implemented AI-driven personalization that analyzes browsing behavior, purchase history, and similar customer profiles to deliver tailored product recommendations, resulting in a 35% increase in average order value.
Operational Efficiency
AI is transforming internal operations across industries:
Predictive Maintenance
Rather than performing maintenance on fixed schedules or waiting for equipment to fail, AI enables predictive maintenance:
- Sensors collect real-time data from equipment
- AI models analyze patterns to predict potential failures
- Maintenance is scheduled precisely when needed
Case Study: A manufacturing company implemented predictive maintenance AI that reduced unplanned downtime by 78% and maintenance costs by 12%, while extending equipment lifespan by an average of 20%.
Intelligent Process Automation
AI-enhanced automation goes beyond traditional robotic process automation (RPA) to handle more complex tasks:
- Document processing and data extraction
- Intelligent routing of inquiries and tasks
- Anomaly detection in processes
- Adaptive workflow optimization
Case Study: A financial services firm implemented intelligent document processing that automatically extracts, validates, and processes information from various document types, reducing processing time from days to minutes and improving accuracy from 82% to 97%.
Decision Support and Analytics
AI is enhancing human decision-making across organizations:
Advanced Analytics and Insights
AI can uncover patterns and insights in data that would be impossible for humans to detect:
- Identifying complex correlations across large datasets
- Generating actionable recommendations
- Providing natural language explanations of data trends
- Creating visual representations of complex information
Case Study: A healthcare provider implemented an AI analytics system that identified previously undetected patterns in patient data, leading to early intervention protocols that reduced hospital readmissions by 28%.
Demand Forecasting
AI has significantly improved the accuracy of demand forecasting:
- Incorporating multiple variables including seasonality, economic indicators, and even weather
- Adapting to changing market conditions
- Providing confidence intervals for predictions
- Enabling scenario planning
Case Study: A retail chain implemented AI-driven demand forecasting that reduced inventory costs by 15% while simultaneously decreasing stockouts by 30%.
Product and Service Innovation
AI is enabling entirely new products and services:
Generative Design
AI can generate numerous design alternatives based on specified constraints:
- Exploring thousands of possible designs
- Optimizing for multiple objectives simultaneously
- Suggesting novel approaches humans might not consider
- Accelerating the design iteration process
Case Study: An automotive manufacturer used generative design AI to create a new vehicle component that is 40% lighter while being 20% stronger than the traditional design, reducing fuel consumption and manufacturing costs.
Intelligent Products
Products themselves are becoming smarter through embedded AI:
- Self-optimizing systems
- Adaptive user interfaces
- Predictive features that anticipate user needs
- Continuous improvement through usage data
Case Study: A smart home device manufacturer embedded AI in their products to learn user preferences and patterns, automatically adjusting settings based on time of day, occupancy, and even individual family members' preferences, resulting in 30% energy savings and significantly higher customer satisfaction.
Implementation Strategies for Business AI
Successfully implementing AI in business requires a strategic approach:
Start with Clear Business Objectives
Effective AI implementation begins with business goals, not technology:
- Identify specific business problems or opportunities
- Define clear success metrics
- Ensure alignment with overall business strategy
- Focus on value creation rather than technology for its own sake
Take an Incremental Approach
Rather than attempting massive AI transformations, successful organizations typically:
- Start with pilot projects that demonstrate value
- Scale gradually based on validated results
- Build on successes and learn from failures
- Develop internal capabilities alongside implementation
Address Data Quality Early
AI systems are only as good as the data they're trained on:
- Audit existing data for quality and completeness
- Implement data governance processes
- Create feedback loops to continuously improve data quality
- Consider data privacy and ethical implications
Focus on Human-AI Collaboration
The most successful AI implementations augment human capabilities rather than replacing them:
- Design AI systems that complement human strengths
- Involve end-users in the design process
- Provide transparency into AI decision-making
- Invest in training and change management
Overcoming Implementation Challenges
Organizations implementing AI typically face several common challenges:
Talent and Expertise
The demand for AI expertise exceeds supply. Successful organizations address this through:
- Building internal capabilities through training and development
- Partnering with specialized AI firms
- Utilizing AI platforms that require less specialized expertise
- Creating cross-functional teams that combine domain and technical knowledge
Integration with Legacy Systems
Many businesses struggle to integrate AI with existing infrastructure:
- Implementing APIs and middleware to connect systems
- Gradually modernizing infrastructure
- Using containerization and microservices architectures
- Creating data lakes to unify access to information
Ethical and Regulatory Considerations
AI implementation must address growing ethical and regulatory concerns:
- Establishing AI ethics committees or review processes
- Implementing bias detection and mitigation
- Ensuring compliance with relevant regulations
- Creating transparent documentation of AI systems
Conclusion
While AI continues to evolve rapidly, businesses don't need to wait for future breakthroughs to derive value from these technologies. Practical applications of AI are already delivering significant benefits across industries, from enhancing customer experiences to optimizing operations and enabling new products and services.
The key to successful AI implementation lies not in chasing the latest technological trends, but in identifying specific business problems where AI can create value, taking an incremental approach to implementation, ensuring data quality, and focusing on human-AI collaboration.
Organizations that approach AI strategically, with a clear focus on business outcomes rather than technology for its own sake, are positioning themselves for competitive advantage in an increasingly AI-driven business landscape.